Algorithms based on artificial intelligence (AI) - supported by increasing digitalization and the Internet of Things (IoT) - enable innovative production solutions to reduce energy consumption in manufacturing. Detailed planning is a particularly suitable area of application.
Energy-efficient production as a competitive factor
For several years now, the focus in the manufacturing industry has been increasingly on ecological criteria. Several developments are accelerating this change. On the one hand, the uncertain supply situation and rising energy costs are forcing companies to reduce their consumption of fossil fuels. On the other hand, there are stricter government regulations with regard to CO2 emissions from industry, such as those set out in the Climate Protection Act. While some are switching to alternative energy sources, according to the VDMA, the primary solution for 85% of companies is to reduce energy consumption. In the long term, innovative approaches to improving energy efficiency are needed to ensure competitiveness in the field of climate-neutral production.
Local approaches to reducing energy consumption have disadvantages
There are a number of approaches to reducing energy consumption in production. They mainly focus on a specific local level and do not take into account the complexity of the production environment. For example, only individual machines are optimized by changing control variables such as processing temperature or working speed. This is often time-consuming and cost-intensive and not scalable. To install a new, energy-saving machine, for example, the production volume has to be reduced or even production stopped completely. Once production is resumed, there is also often a temporary drop in production quality.
AI-supported detailed planning as a possible solution
The use of AI-based solutions in detailed planning, on the other hand, makes it possible to achieve energy savings in production without having to intervene in the production processes. The production steps are distributed to machines in such a way that energy consumption is minimized. On the one hand, AI algorithms can take into account the energy consumption of a machine when processing a production step. On the other hand, it takes into account whether energy-intensive switch-on and switch-off processes or inefficient idle times occur when using a machine. This is particularly important as energy consumption during non-working time - i.e. during idle times or switch-on and switch-off processes - can account for up to 65% of the total consumption of a production process.
Production situations with significantly high energy consumption for starting up and shutting down machines therefore offer great potential for savings. They are particularly suitable for optimization with artificial intelligence. To avoid the energy-intensive start-up of a machine and long idle intervals, a production step is assigned to a machine that is already running, for example. By intelligently sequencing production steps and simultaneously selecting particularly energy-saving machines, the total energy consumption of a production process can be reduced by up to 20% (see Fig. 1).
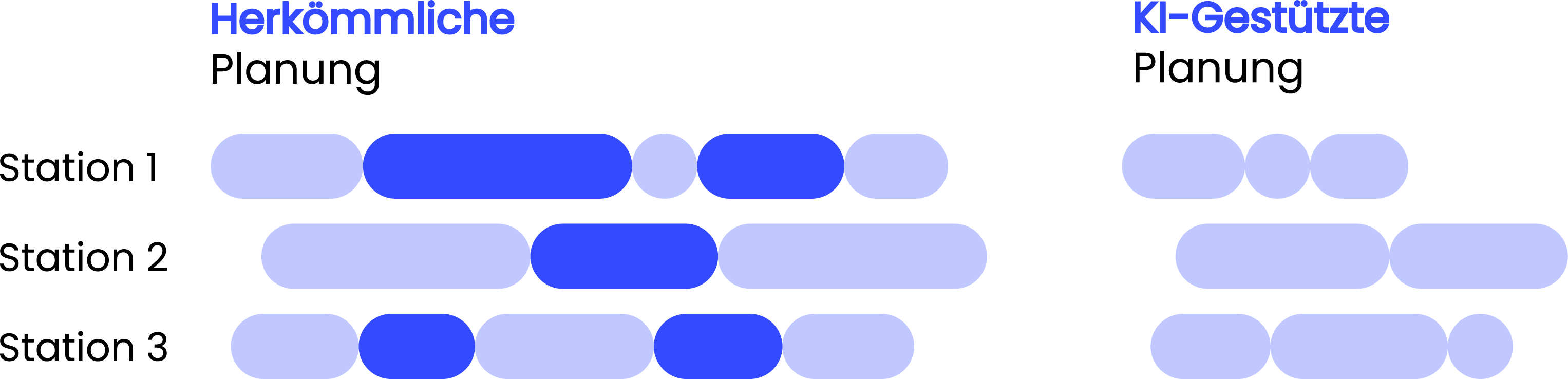
Ecological vs. economic conflicts of objectives in detailed planning
Forefficient production, economic targets must also be taken into account in production planning alongside ecological target criteria such as energy consumption. One relevant target criterion from an economic perspective is on-time delivery (or total production time). To optimize on-time delivery, it often makes sense to use as many machines as possible in order to be able to produce very quickly. However, this can lead to many energy-intensive startup and shutdown processes. From an energy efficiency perspective, it can therefore make more sense to produce on fewer machines, but for longer (see Figure 2). While one might intuitively assume that a production schedule that is as close to the delivery date as possible - i.e. short - also consumes very little energy, this is not always the case.

It is therefore necessary to weigh up the two criteria. An AI solution can implement this independently and thus generate a balanced solution. In addition, several "equally good" plans can be generated that focus more on either energy consumption or adherence to delivery dates. These plans can serve as support for planners who, based on expert knowledge, can select the optimum production plan for the current situation from the set of all plans generated (see Figure 3).
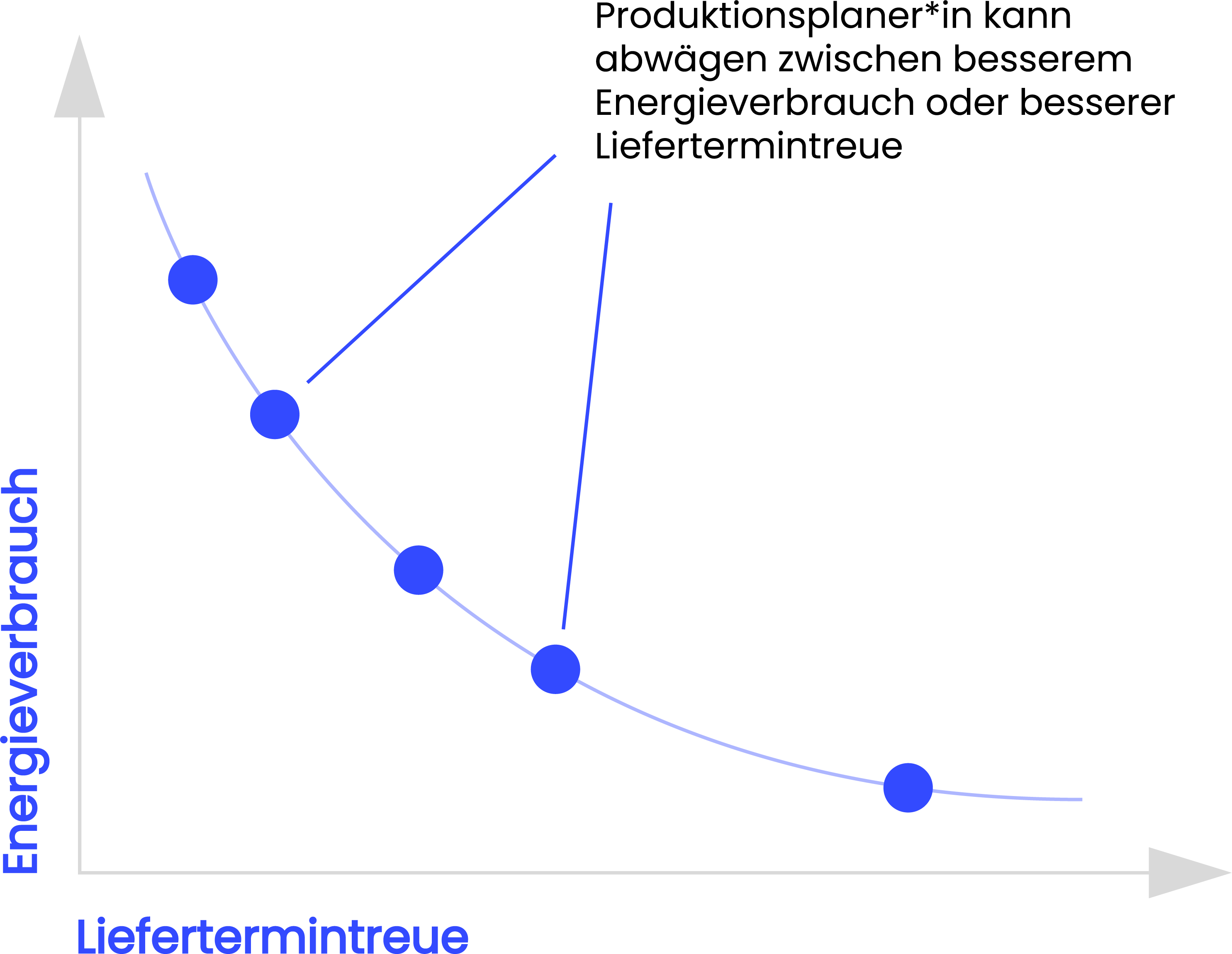